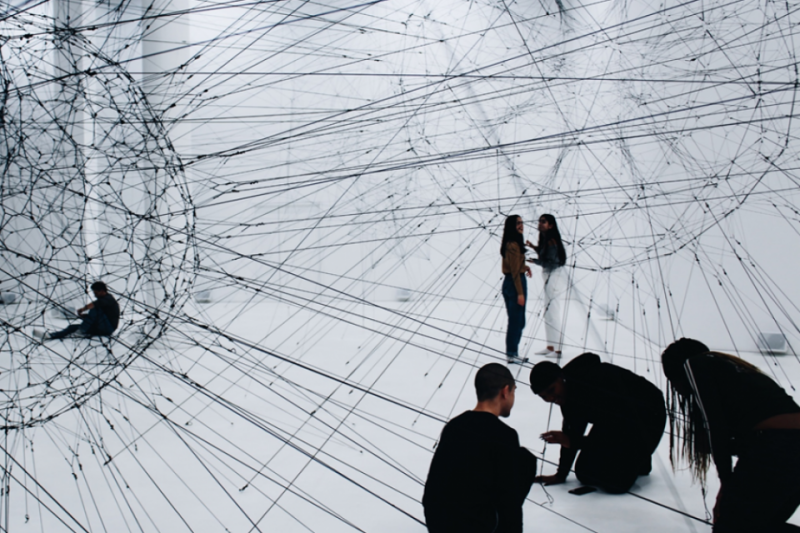
Electronic health records (EHRs) comprise a remarkably rich source of longitudinal health data. For every health system, within each patient’s EHR are data compiled from across multiple domains, including demographics, diagnoses, medications, and detailed records for any encounters with primary care, specialty care, emergency room visits, and hospital stays. At a large institution such as Duke Health where care has been rendered for millions of patients across the years, the EHR presents a vast and alluring “big data” resource for researchers who want to mine it in pursuit of answers to a virtually limitless number of questions.
The potential of this expanse of EHR data offered a siren song for our team when we embarked on a Forge demonstration project in late 2018. As part of a multidisciplinary group of clinicians, informaticists, policy analysts, biostatisticians, and computer scientists, we have been investigating whether we can apply machine learning methods to EHR data to develop models that accurately predict risk for autism spectrum disorder (ASD) and attention deficit/hyperactivity disorder (ADHD). The impact of a successful model is potentially enormous, because while these two conditions emerge early in life, are associated with a wide range of comorbidities, and are predictive of many adverse outcomes later in life, they also respond well to early interventions that can meaningfully reduce the risk of those adverse outcomes.
We started our work by building a cohort of patients from the Duke EHR that we could use to perform retrospective analyses and develop predictive models that we hope to prospectively validate in the context of other ongoing NIH-funded projects—an idea initially conceived as a Design Workshop proposal and supported by Duke Forge.
We defined our cohort as all children born in the Duke University Health System over 10 years (from 2006 to 2016; approximately 200,000 total). This enabled us to use data from at least the first three years of life for all of children in the cohort. To get started with our analyses and modeling, we sought demographic information, diagnosis codes, and procedure codes, including for primary and specialty care, outpatient clinic visits, emergency department encounters, and inpatient hospital admissions. At the outset, our clinical project leads expected this step to be a simple task—after all, we knew from experience that all of this information is recorded in detail in the EHR—and then we could quickly proceed to higher-order modeling.
We quickly learned how naïve our initial assumptions of simplicity were. As our project has progressed, the process of developing a meaningful predictive model has been both challenging and rewarding—and our work continues. The following are some of the most salient lessons.
EHR data are messy and not necessarily organized in ways clinicians and clinical researchers might expect.
Many clinicians, even (or especially) those familiar with EHRs in their day-to-day clinical practice, who are interested in conducting research with EHR data might assume that extracting the data they need to answer research questions is a relatively simple querying task. It is most certainly not! The clinical outcomes, measures, and variables that seem intuitive to clinicians may be captured and structured in myriad complex ways in the EHR. This is not altogether surprising. Clinical researchers are accustomed to working with highly curated datasets that were rigorously collected and stored in databases expressly designed to support detailed analytical plans, such as those needed for clinical trials.
Electronic health records, on the other hand, are typically designed for very different purposes: to support the delivery of care to patients and billing for that care. This means that the structure and organization of EHR data is very different compared with a clinical trial’s analytical dataset. When using EHR data to answer clinical research questions, we quickly learned what a difficult and daunting task it is to distill highly complex and often disorganized EHR data into meaningful elements that can be used for research.
EHR data are often incomplete.
While the Duke Health EHR contains reliable and detailed records for care received in the Duke Health system or associated practices, it may be missing records for care received outside of the system. A child’s emergency room visit at UNC Hospital, an urgent care encounter while on vacation in Florida, or a visit with a specialist in Charlotte—any of these health encounters might be missing from the Duke EHR. Additionally, many patients come to Duke only for specialist visits and receive their primary care outside of the Duke system, leaving those records inaccessible and potentially skewing the data. In the context of our project, these missing data may include important predictors that we want to include in our modeling. Although we know that the record is likely incomplete in nonrandom ways, we don’t have a good way to estimate or account for what is missing.
Information that is easily accessed through the front end of the EHR is not easily extracted through the back end.
This reality makes data-related questions particularly nonintuitive for clinicians who are familiar with the front end of the EHR system. For example, a clinician may be able to easily navigate a single patient’s record to locate a piece of information, but if that data element is contained in a scanned document, clinical note narrative, or other unstructured data field, then it’s not easy to extract, process, and analyze through the back end of the EHR—something that’s essential when operating on a scale of hundreds of thousands of patients.
To further complicate this process, clinical data in the EHR may be recorded in multiple places. For example, the date a diagnosis is made could be a critical data point in a research study. In the EHR, that diagnosis may be captured in multiple places, including the diagnosis list, but also in the problem list and in clinical note narratives, and not all appearances of the diagnosis would necessarily be associated with the same date. In such cases, the EHR does not lend itself easily to querying this seemingly basic information, so researchers must carefully define what they mean by “date of diagnosis” in the context of the EHR data.
Another challenge related to incompleteness is that data that are obvious or easy to gather clinically, such as information about a child’s parents, is not easily linked in the EHR. For the purposes of our study, many of the risk factors and potential predictors for ASD and ADHD could be gleaned from the health records of patients’ parents. Although in many instances those health records are contained in the Duke Health EHR, those records are not automatically joined in a way that facilitates aggregating and extracting them together for analysis.
There are differences in the accessibility of data before and after the implementation of a single, integrated EHR at Duke.
The implementation of system-wide Epic EHR software was an important change that occurred within Duke Health during the timeframe we examined in our cohort. Although patient records were being recorded in electronic format prior to the rollout of Epic, they were Balkanized across departments and practices instead of residing under a single system. For example, in the Department of Psychiatry, health records were gathered via a system only used for that department. Due to this segmentation of records prior to the transition to a system-wide integration, our team has had to carefully consider which data elements are reliable in our cohort across the boundary from pre- to post-Epic implementation.
To be certain, the seemingly simple first step of extracting the EHR data for our cohort has taken substantially more time and effort than we first anticipated. However, working through this process and addressing these challenges has proved to be an extremely valuable experience for our team. To productively harness EHR data for research, it takes time and effort to really understand that data. This requires pressure testing, manually checking for verification, and quite often having your assumptions blown up in frustrating fashion. Success requires having the right people involved, including a mix of clinical and quantitative experts, to ensure that data are being used and interpreted properly.
We’re convinced that the progress we’ve made has only been possible thanks to the team-based approach we took for this project, with clinicians working closely with our quantitative experts. This key lesson learned should be a foundational tenet, not just for ourselves and our colleagues at the Duke Forge, but for anyone seeking to bring health data science to scale and unlock the vast potential of EHRs. As we advance into the era of learning health systems, we need to systematize a process for how clinicians and data scientists can work together to solve important problems with EHR data. Otherwise we will struggle with the inefficiency of every new team having to learn the same lessons over and over again.
Learning through these obstacles has provided our team with an avenue for transforming ourselves and our capacities. Because we gained so much experience confronting these challenges faced when working with EHR data, we better understand its opportunities, limitations, and idiosyncrasies. The slope of our learning curve has changed dramatically, and we are now better able to leverage the EHR data to examine not only the question we started with, but many more that we will investigate in the future.
This article was originally published on the Duke Forge website and was republished with permission.
Andrew Olson, MPP, Duke Forge's Associate Director for Policy Strategy and Solutions for Health Data Science, is a health policy specialist and experienced project leader. In his role with the Forge, he helps develop demonstration projects and other initiatives that address or inform critical health policy issues, and facilitates the translation of health data science discoveries to a policy audience.
Scott Kollins, PhD, is a professor in the Department of Psychiatry and Behavioral Science the Duke University School of Medicine. A clinical psychologist, his research interests include psychopharmacology and the intersection of ADHD and substance abuse. Dr. Kollins is the Global Lead for ADHD and Substance Use Disorders at the Duke Clinical Research Institute (DCRI). He is also the Director of the Duke ADHD Program.
You can find out more about Dr. Kollins' work in this article from the Duke School of Medicine's online magazine, Duke Magnify: "Harnessing the Power of Machine Learning for Earlier Autism Diagnosis."